Uniquely positioned to help you navigate market uncertainty
Uniquely positioned to help you navigate market uncertainty
Each situation is unique, each client is different, and the marketplace is always changing. One constant is our goal to offer the best possible advice, tools and execution to help you succeed – whatever your goals.
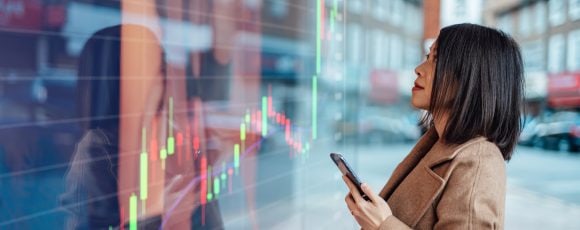
UBS Neo
UBS Neo
Built to seamlessly optimize the way you work; from traders looking to access liquidity and execute across asset, to Portfolio Managers consuming research and market expertise across our global trading floors. UBS Neo is an open-platform and firmwide ecosystem that empowers UBS communities to create, discover and consume investment capabilities via mobile, desktop, web and API channels.
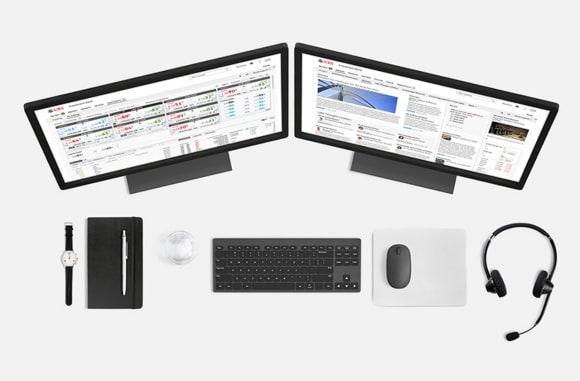
Best Global Bank for Financial Institutions
Best Global Bank for Financial Institutions
Global Finance, 2023
Best House, Structured Retail Products, Switzerland
Best House, Structured Retail Products, Switzerland
SRP Europe Awards, 2023
Top 3 Global Equity Research Team
Top 3 Global Equity Research Team
Institutional Investor, 2016 - 2023
We believe our culture is the single most important factor to our success. It shapes policy and processes, informs what it is like to work here, and delivers long-term value to our stakeholders. Everyone from our President to our newest employees are equally responsible for owning and protecting our culture, and everyone can influence it.
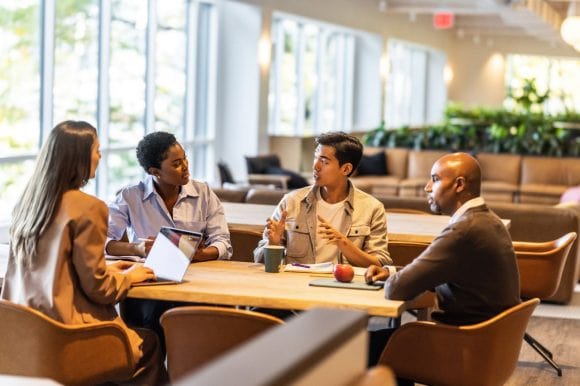
Fraud Alert
Fraud Alert
Please be aware that the integration of Credit Suisse and UBS creates a unique opportunity for fraudsters to contact our clients purporting to be from our organisation or selling fake investment schemes from our entities. This could be a means to get information from our clients or to trick clients into sending money to a new account. Please be vigilant if you are contacted by an unknown party. UBS and Credit Suisse entities will never contact you by email or telephone with new bank account details. Before transferring money or sharing personal details, please contact UBS (or relevant bank) by telephone. If you are already a client, please contact your UBS or Credit Suisse advisor.
Further advice on staying safe online can be found at www.ubs.com/cybersafe.