Twice a year, our senior investment leadership discusses the long- and short-term themes that are likely to move markets over the next year.
We offer global scale, breadth and depth of solutions to help investors build fully diversified portfolios.
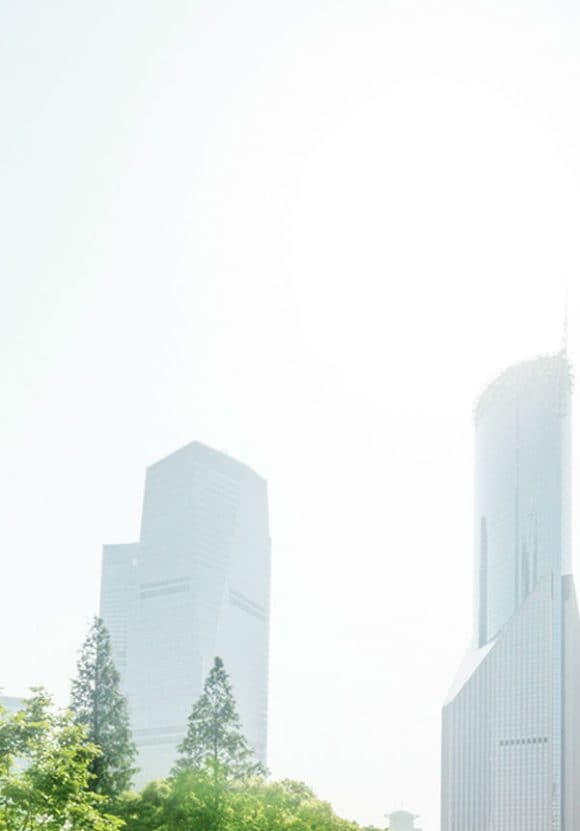
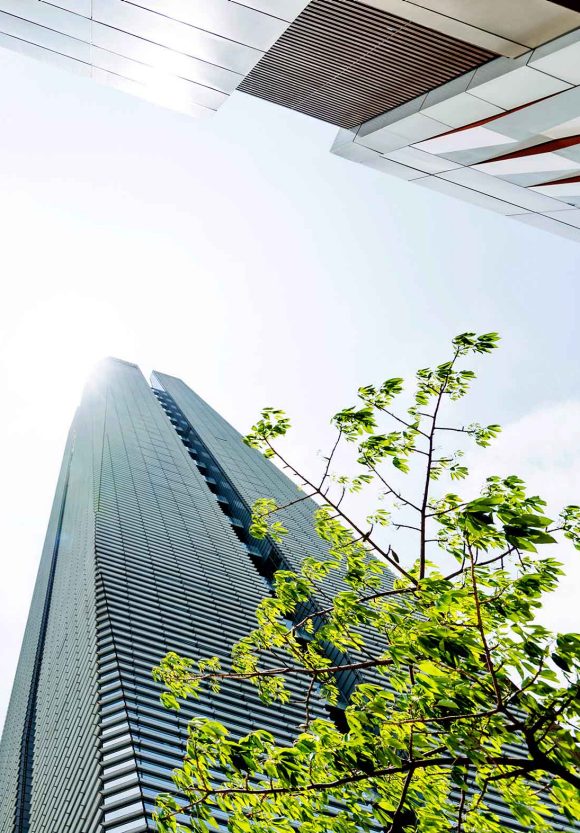
header.search.error
Twice a year, our senior investment leadership discusses the long- and short-term themes that are likely to move markets over the next year.
We offer global scale, breadth and depth of solutions to help investors build fully diversified portfolios.